Everything changed as soon as the world realized generative AI was no longer the stuff of fantasy.
However, large language models, the technology powering generative AI, aren’t without their limitations.
Because they’re restricted to the datasets they were trained on, they can’t give up-to-date responses, struggle to understand the context of what’s being asked, and sometimes guess at or hallucinate answers.
Retrieval Augmented Generation changes that give AI applications the benefits of specific and current information.
Canadian Lawyer Mag recently reported on how this is disrupting the legal industry, and the tech industry is not far behind.
Leveling The Playing Field
In the long run, the biggest impact of RAG might well be how effectively it levels the industry’s playing field. RAG supercharges the productivity of developers, marketers, customer service executives, and even CEOs, allowing small startups to operate at the same level as massive organizations.
Rapid And Accurate Answers
Because RAG allows LLMs to combine specialized and specific knowledge bases with their training data, it makes applications much better at answering questions.
This can be a game changer in the tech industry, where competitive edges are often found in being able to spot innovative solutions in a sea of technical details.
Information retrieval
Not only does RAG make LLMs better at providing useful answers, it also makes them better at finding answers.
RAG helps to add more context for LLMs to use when trying to understand what the user really wants, and this, in turn, means it’s able to fetch information that is helpful instead of just in the right area.
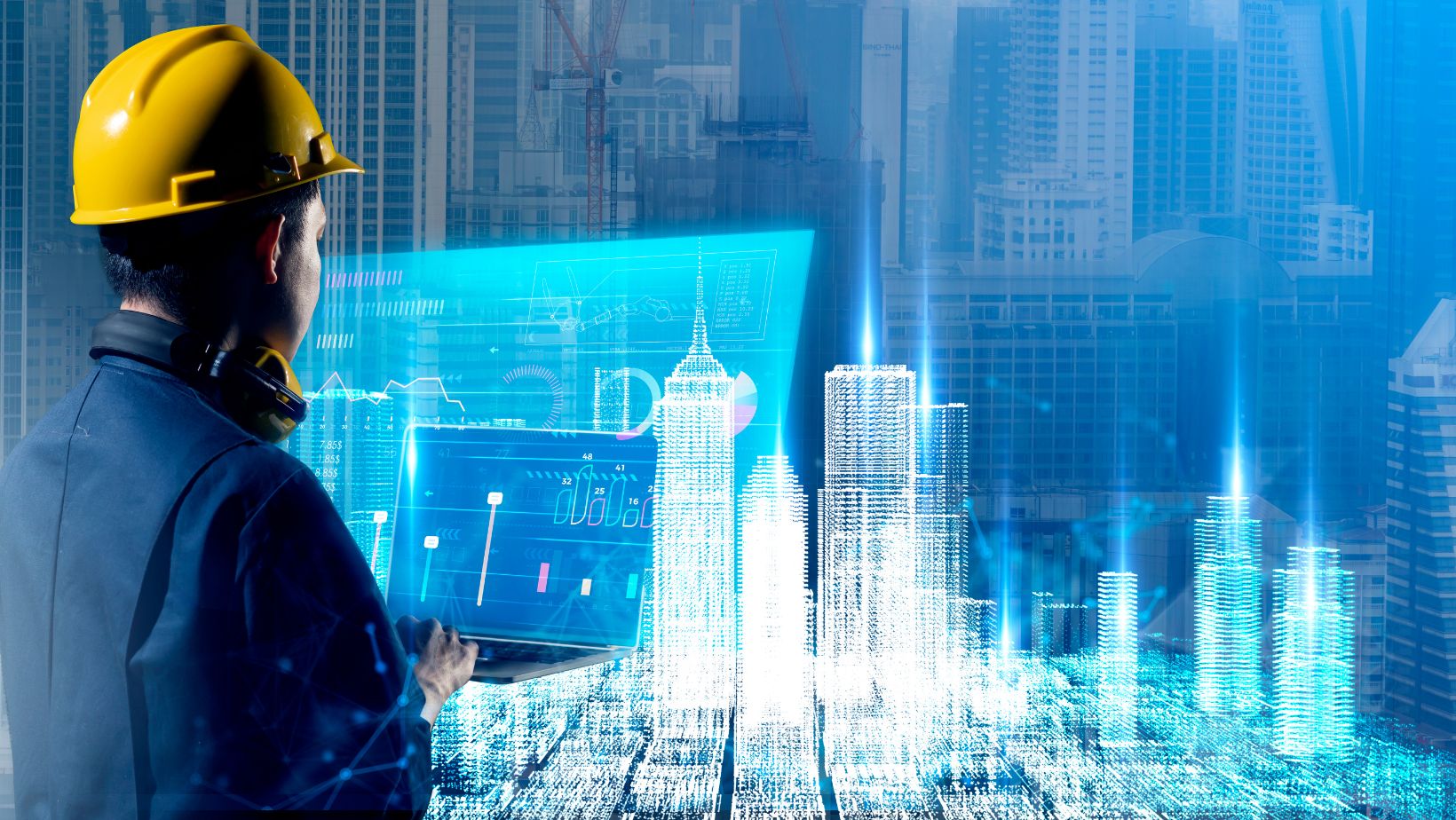
As a post by MongoDB about retrieval augmented generation explains, “To make an LLM useful for your specific generative AI-powered application or project, you need to make sure you are feeding it with your own relevant data.”
Coding Assistants
The arrival of RAG also signals the arrival of genuine AI assistants and could take productivity to the next level, as DevOps’ article on the rise of coding assistants explains.
Because RAG can use real-time user data to supplement the LLM’s knowledge base, a developer’s live actions can be used to augment a prompt so that the AI application is able to follow along and offer useful suggestions.
“Why doesn’t my code work?” suddenly becomes a question you can ask with the genuine hope of a useful answer.
Data Analysis
The tech industry has become more and more competitive.
This has led to an intensely increasing obsession with data analysis, looking for patterns and trends that will help organizations understand their customers, their markets, and their own products.
RAG and generative AI significantly improve this, allowing companies to rapidly assess the market they’re working in, and even leverage predictive analytics to work out what their potential customers want before they want it.
Risk Management
Live information from sources like the stock exchange and company-specific documentation, both knowledge sources that would be beyond an LLM but not one paired with RAG, can offer sophisticated risk management solutions.
This means the application can consider not only what has happened historically in similar situations or what is happening right now on the stock market but also what has happened previously to your company and how your company plans to work.
Personalized Solutions
One of the greatest benefits of RAG is that it allows for real personalization without the data security worries.
Without RAG the idea of fine-tuning an LLM, especially one that remains owned by its inventors, with personal data would’ve been an immediate red flag for anyone remotely concerned with keeping their data secure in the digital world.
RAG allows this data to be used securely by the LLM so that the generative AI application can offer responses that consider who the user is as an individual.
Recommendations You Care About
Because RAG can leverage specific knowledge bases, and even information about the users themselves, it can give suggestions and recommendations that will actually make sense and help in specific use cases.
So, when you ask, “what’s the best database solution for this?” you get a response that understands how you work, and what you’re trying to achieve.
To understand more about quite how much of an impact this is all going to have, check out our post on the ‘Impact of Generative AI Tools on Modern Work.’
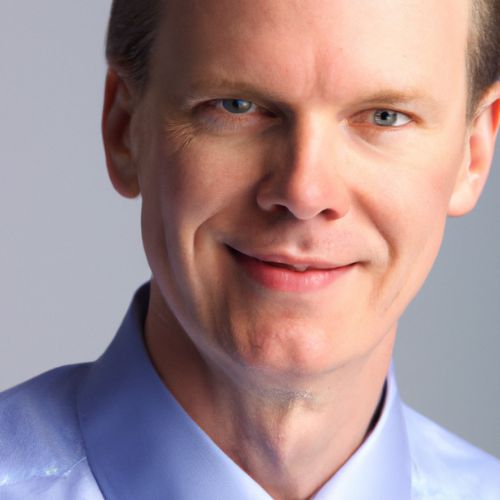